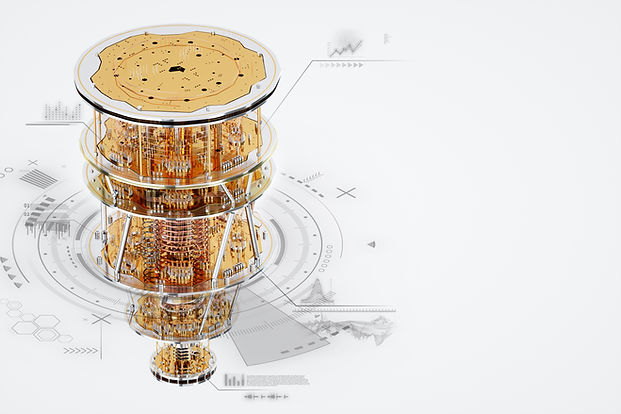
Next-Generation Credit Risk Modeling : From IRB and IFRS 9 to Quantum ML
COURSE OBJECTIVE
Advanced Credit risk modeling course using Artificial Intelligence and Quantum Computing, among many other topics: credit scoring tools, modeling of PD, LGD and EAD parameters of the advanced IRB approach of Basel III, credit risk methodologies for IFRS 9 impairment models, stress testing models of credit risk, economic capital and climate change-related credit risk.
Among other topics, quantum computing, quantum circuits, important quantum algorithms, quantum mechanics, quantum error and correction, and quantum machine learning are exposed.
Machine and deep learning are used to build powerful credit scoring and behavior scoring tools, as well as to estimate and calibrate risk parameters and stress testing.
A module on advanced data processing is exposed, explaining among other topics: sampling, exploratory analysis, outlier detection, advanced segmentation techniques, feature engineering and classification algorithms.
The course explains the recent final reforms of Basel III regarding the new standard approach and Advanced IRB, IFRS 9 related to credit risk and the new guidelines on estimation of PD and LGD and treatment of exposures in default of EBA.
Predictive machine learning models are shown such as: decision trees, neural networks, Bayesian networks, Support Vector Machine, ensemble model, etc. And in terms of neural networks, feed forward, recurrent RNN, convoluted CNN and adversarial Generative architectures are exposed. In addition, Probabilistic Machine Learning models such as Gaussian processes and Bayesian neural networks have been included.
Advanced methodologies are taught to estimate and calibrate risk parameters: PD, LGD and EAD. The Lifetime PD estimate used in the IFRS 9 impairment models is exposed.
Methodologies and practical exercises of Stress testing in credit risk using advanced techniques of machine learning and deep learning are shown. And a practical exercise with financial statements to understand the impact of stress testing on capital and profits.
We have a global exercise to estimate the expected loss at 12 months and ECL lifetime using advanced credit risk methodologies, including PD, LGD, EAD, prepayment and interest rate curve models.
The course teaches about economic capital methodologies that are used in credit card, mortgage, SME, and corporate portfolios. It also covers capital allocation methodologies.
Quantum Machine Learning is a fusion of quantum algorithms with Machine Learning programs. Machine learning algorithms are used to process huge amounts of data, whereas quantum machine learning employs qubits and specialized quantum systems to enhance the speed of computation and data storage in an algorithm. For instance, some mathematical and numerical techniques from quantum physics can be used in classical deep learning. A quantum neural network has the potential to reduce the number of steps, qubits used, and computation time.
The aim of this course is to demonstrate how quantum computing and tensor networks can be utilized to enhance the accuracy of machine learning algorithms. We will showcase how quantum algorithms can expedite Monte Carlo simulations, which are the most potent tools for constructing credit risk models. This provides a significant advantage for calculating economic capital, lifetime PD, and creating stress testing scenarios.
The course also seeks to compare classical models with quantum models, and make clear the scope, benefits, and opportunities of quantum computing in this field.
WHO SHOULD ATTEND?
The course is designed for financial professionals who want to enhance their skills in developing effective credit scoring models and fine-tuning their results. It is also suitable for credit risk and data science department managers responsible for managing these models. To better comprehend the course topics, participants are required to have a solid foundation in mathematics and statistics. You can benefit from quantum computing technologies without needing to have knowledge of quantum physics.
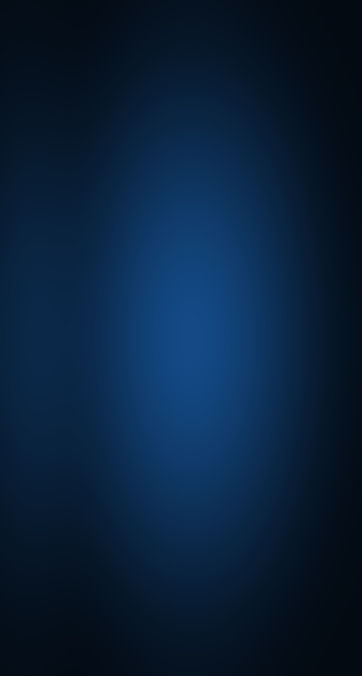

Schedules:
-
Europe: Mon-Fri, CEST 16-19 h
-
America: Mon-Fri, CDT 18-21 h
-
Asia: Mon-Fri, IST 18-21 h

Price: 7 900 €

Level: Advanced

Duration: 39 h

Material:
-
Presentations PDF
-
Exercises in Excel, R, Python, Jupyterlab y Tensorflow
1. Cutting-Edge Techniques Integration: The course offers a unique blend of traditional credit risk modeling methodologies with cutting-edge technologies such as artificial intelligence and quantum computing. By incorporating advanced techniques like quantum machine learning and deep learning, participants gain a comprehensive understanding of modern risk assessment methodologies, equipping them with the skills needed to tackle complex credit risk scenarios in the era of digital transformation.
2. Enhanced Accuracy and Efficiency: Through the utilization of quantum algorithms and tensor networks, participants learn how to enhance the accuracy and efficiency of credit risk models. Quantum computing enables expedited Monte Carlo simulations, a crucial component in constructing robust credit risk models, thereby providing a significant advantage in calculating economic capital, lifetime PD, and developing stress testing scenarios. This enhanced computational capability empowers financial institutions to make more informed decisions and better manage their credit risk exposure.
3. Preparation for Future Challenges: By exploring topics such as climate change-related credit risk and the latest reforms in Basel III and IFRS 9, participants are equipped with the knowledge and skills needed to navigate evolving regulatory landscapes and emerging risk factors. Additionally, exposure to quantum computing and its potential applications in credit risk modeling prepares participants for the future of finance, ensuring they remain at the forefront of innovation in the field. This forward-looking approach enables financial professionals to anticipate and address future challenges effectively, thereby enhancing the resilience and sustainability of their organizations.
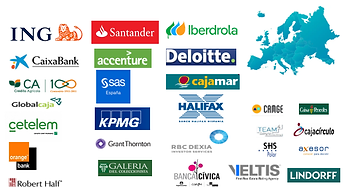
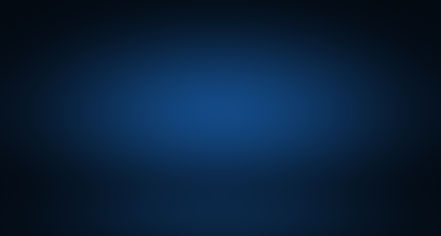
AGENDA
Next-Generation Credit Risk Modeling :
From IRB and IFRS 9
to Quantum ML
PD, LGD and EAD IRB
Module 1: Guidelines on PD estimation, LGD estimation and the treatment of defaulted exposures
-
Reduction of parameter variability
-
Homogenization of the calculation of PD and LGD
-
Implementation dates in European banks
-
Data quality
-
Representativeness of data for model development and for risk parameter calibration
-
Human judgement in estimation of risk parameters
-
Deficiency treatment and margin of conservatism (Moc)
-
PD estimation
-
Model development
-
Data requirements
-
Risk drivers and rating criteria
-
Processing of external ratings
-
Rating philosophy
-
Treatment of Pools
-
-
PD calibration
-
Data requirements
-
Calculation of one-year default rate
-
Calculation and use of observed average default rate
-
Long-run average default rate
-
Long-run average default rate calibration
-
-
LGD estimation
-
Methodologies for PD estimation
-
Data requirements
-
Recoveries from collaterals
-
Model development
-
Risk drivers
-
Collateral eligibility
-
Collateral inclusion
-
-
LGD Calibration
-
Definition of economic loss and realized loss
-
Treatment of commissions, interest and other withdrawals after default
-
Discount rate
-
Direct and indirect costs
-
Long-run average LGD
-
Calibration of the estimates to long-run average LGD
-
-
Estimation of risk parameters for defaulted exposure
- Estimation and calibration of the Expected Loss Best Estimate
-
Estimation and calibration of LGD in-default
-
Application of risk parameters
-
Review of estimates
-
Accompanying documents
-
Impact assessment
-
Problem identification
-
Policy objectives
-
Baseline Scenario
-
Options considered
-
Cost-benefit analysis
IFRS 9
Module 2: Implementation of IFRS 9 in the EU
-
SICR assessment approaches
-
Approaches for determining stage transfers
-
Alignment between the Definition of Default and IFRS 9 exposures in Stage 3
-
Low credit risk exemption
-
12-month PD as proxy for lifetime PD
-
-
Expected Credit Loss Models
-
Types of Expected Credit Loss models
-
Model limitations and use of overlays
-
Effects from the Russian/Ukrainian conflict
-
ESG including climate risks
-
-
IFRS 9 PD variability and robustness
-
Variability in the IFRS 9 PD
-
Differences in the use of IRB models for IFRS 9 estimates
-
Differences in the definition of default
-
Differences in risk differentiation
-
Differences in risk quantification
-
Treatment of 2020-2021 defaults
-
-
Incorporation of forward-looking information
-
Macroeconomic scenarios
-
Variability of the methodological approach for incorporation of FLI and
reflection of non-linearity-
Incorporation of FLI at parameter level
-
List of macroeconomic variables used for FLI incorporation
-
Forecasting period and reversion to long-term average
-
-
Variability in the impact and different sensitivities from FLI
-
Effect of non-linearity and probability framework
-
-
Focus on backtesting practices
-
Staging allocation
-
ECL measurement
-
IFRS 9 LGD estimates
-
IFRS 9 PD estimates
-
Overlays
-
Forward-looking information
-
CREDIT SCORING
Module 3: Exploratory Analysis
-
Exploratory Data Analysis EDA
-
Data sources
-
Data review
-
Target definition
-
Time horizon of the target variable
-
Sampling
-
Random Sampling
-
Stratified Sampling
-
Rebalanced Sampling
-
-
Exploratory Analysis:
-
Histograms
-
Q Q Plot
-
Moment analysis
-
boxplot
-
-
Treatment of Missing values
-
Multivariate Imputation Model
-
-
Advanced Outlier detection and treatment techniques
-
Univariate technique: winsorized and trimming
-
Multivariate Technique: Mahalanobis Distance
-
-
Over and Undersampling Techniques
-
Random oversampling
-
Synthetic minority oversampling technique (SMOTE)
-
Module 4: Feature engineering
-
Feature engineering
-
Data Standardization
-
Variable categorization
-
Equal Interval Binning
-
Equal Frequency Binning
-
Chi-Square Test
-
-
Binary coding
-
WOE Coding
-
WOE Definition
-
Univariate Analysis with Target variable
-
Variable Selection
-
Treatment of Continuous Variables
-
Treatment of Categorical Variables
-
Information Value
-
Optimization of continuous variables
-
Optimization of categorical variables
-
-
Exercise 1: EDA Exploratory Analysis
-
Exercise 2: Feauture Engineering
-
Exercise 3: Detection and treatment of Advanced Outliers
-
Exercise 4: Multivariate model of imputation of missing values
-
Exercise 5: Univariate analysis in percentiles in R
-
Exercise 6: Continuous variable optimal univariate analysis in Excel
Machine Learning
Module 5: Machine Learning
-
Unsupervised models
-
K Means
-
Principal Component Analysis (PCA)
-
Advanced PCA Visualization
-
Supervised Models
-
Ensemble Learning
-
Bagging trees
-
Random Forest
-
Boosting
-
Adaboost
-
Gradient Boosting Trees
-
Exercise 7: Segmentation data using K-Means
-
Exercise 8: Credit Scoring Support Vector Machine
-
Exercise 9: Credit Scoring Boosting
-
Exercise 10: Credit Scoring Bagging
-
Exercise 11: Credit Scoring Random Forest, R and Python
-
Exercise 12: Credit Scoring Gradient Boosting Trees
DEEP LEARNING
Module 6: Deep Learning
-
Feed Forward Neural Networks
-
Single Layer Perceptron
-
Multiple Layer Perceptron
-
Neural network architectures
-
Activation function
-
Back propagation
-
Directional derivatives
-
Gradients
-
Jacobians
-
Chain rule
-
Optimization and local and global minima
-
-
Deep Learning Convolutional Neural Networks CNN
-
CNN for pictures
-
Design and architectures
-
Convolution operation
-
Filters
-
Strider
-
Padding
-
Subsampling
-
Pooling
-
Fully connected
-
Credit Scoring using CNN
-
Recent CNN studies applied to credit risk and scoring
-
-
Deep Learning Recurrent Neural Networks RNN
-
Long Term Short Term Memory (LSTM)
-
Hopfield
-
Bidirectional associative memory
-
descending gradient
-
Global optimization methods
-
RNN and LSTM for credit scoring
-
One-way and two-way models
-
-
Generative Adversarial Networks (GANs)
-
Generative Adversarial Networks (GANs)
-
Fundamental components of the GANs
-
GAN architectures
-
Bidirectional GAN
-
Training generative models
-
Credit Scoring using GANs
-
-
Exercise 14: Credit Scoring using Deep Learning Feed Forward
-
Exercise 15: Credit scoring using deep learning CNN
-
Exercise 16: Credit Scoring using Deep Learning LSTM
-
Exercise 17: Credit Scoring using GANs
Module 7: Tuning Hyperparameters in Deep Learning
-
Hyperparameterization
-
Grid search
-
Random search
-
Bayesian Optimization
-
Train test split ratio
-
Learning rate in optimization algorithms (e.g. gradient descent)
-
Selection of optimization algorithm (e.g., gradient descent, stochastic gradient descent, or Adam optimizer)
-
Activation function selection in a (nn) layer neural network (e.g. Sigmoid, ReLU, Tanh)
-
Selection of loss, cost and custom function
-
Number of hidden layers in an NN
-
Number of activation units in each layer
-
The drop-out rate in nn (dropout probability)
-
Number of iterations (epochs) in training a nn
-
Number of clusters in a clustering task
-
Kernel or filter size in convolutional layers
-
Pooling size
-
batch size
-
Interpretation of the Shap model
-
Exercise 18: Tuning hyperparameters in Xboosting, Random forest and SVM models for credit scoring
-
Exercise 19: Tuning hyperparameters in Deep Learning model for credit scoring
Module 8: The development process of the scorecard
-
Scoring assignment
-
Scorecard Classification
-
Scorecard WOE
-
Binary Scorecard
-
Continuous Scorecard
-
-
Scorecard Rescaling
-
Factor and Offset Analysis
-
Scorecard WOE
-
Binary Scorecard
-
-
Reject Inference Techniques
-
Cut-off
-
Parceling
-
Fuzzy Augmentation
-
Machine Learning
-
-
Advanced Cut Point Techniques
-
Cut-off optimization using ROC curves
-
-
Exercise 20: Creating a scorecard using Excel, R, and Python
QUANTUM COMPUTING
Module 9: Quantum Computing and Algorithms
-
Future of quantum computing in banking
-
Is it necessary to know quantum mechanics?
-
QIS Hardware and Apps
-
Quantum operations
-
Qubit representation
-
Measurement
-
Overlap
-
Matrix multiplication
-
Qubit operations
-
Multiple Quantum Circuits
-
Entanglement
-
Deutsch Algorithm
-
Quantum Fourier transform and search algorithms
-
Hybrid quantum-classical algorithms
-
Quantum annealing, simulation and optimization of algorithms
-
Quantum machine learning algorithms
-
Exercise 21: Quantum operations
QUANTUM MACHINE LEARNING
Module 10: Development of Credit Scoring using Quantum Machine Learning
-
What is quantum machine learning?
-
Qubit and Quantum States
-
Quantum Automatic Machine Algorithms
-
Quantum circuits
-
K means quantum
-
Support Vector Machine
-
Support Vector Quantum Machine
-
Variational quantum classifier
-
Training quantum machine learning models
-
Quantum Neural Networks
-
Quantum GAN
-
Quantum Boltzmann machines
-
Quantum machine learning in Credit Risk
-
Quantum machine learning in credit scoring
-
Quantum software
-
Exercise 22: Quantum Support Vector Machine to develop credit scoring model
-
Exercise 23: Quantum feed forward Neural Networks to develop a credit scoring model and PD estimation
-
Exercise 24: Quantum Convoluted Neural Networks to develop a credit scoring model and PD estimation
Module 11: Tensor Networks for Machine Learning
-
What are tensor networks?
-
Quantum Entanglement
-
Tensor networks in machine learning
-
Tensor networks in unsupervised models
-
Tensor networks in SVM
-
Tensor networks in NN
-
NN tensioning
-
Application of tensor networks in credit scoring models
-
Exercise 25: Construction of credit scoring and PD using tensor networks
PROBABILISTIC MACHINE LEARNING
Module 12: Probabilistic Machine Learning
-
Probability
-
Gaussian models
-
Bayesian Statistics
-
Bayesian logistic regression
-
Kernel family
-
Gaussian processes
-
Gaussian processes for regression
-
-
Hidden Markov Model
-
Markov chain Monte Carlo (MCMC)
-
Metropolis Hastings algorithm
-
-
Machine Learning Probabilistic Model
-
Bayesian Boosting
-
Bayesian Neural Networks
-
Exercise 26: Gaussian process for regression
-
Exercise 27: Bayesian neural networks
ADVANCED VALIDATION
Module 14: Advanced Validation of AI Models
-
Integration of state-of-the-art methods in interpretable machine learning and model diagnosis.
-
Data Pipeline
-
Feature Selection
-
Black-box Models
-
Post-hoc Explainability
-
Global Explainability
-
Local Explainability
-
Model Interpretability
-
Diagnosis: Accuracy, WeakSpot, Overfit, Reliability, Robustness, Resilience, Fairness
-
Model comparison
-
Comparative for Regression and Classification
-
Fairness Comparison
-
-
Exercise 28: Validation and diagnosis of advanced credit scoring models
IRB PD
Module 15: Probability of Default PD IRB
-
PD estimation
-
Econometric models
-
Machine Learning Models
-
Data requirement
-
Risk drivers and credit scoring criteria
-
Rating philosophy
-
Pool Treatment
-
-
PD Calibration
-
Default Definition
-
Long run average for PD
-
Technical defaults and technical default filters
-
Data requirement
-
One Year Default Rate Calculation
-
Long-Term Default Rate Calculation
-
-
PD Model Risk
-
Conservatism Margin
-
-
PD Calibration Techniques
-
Anchor Point Estimate
-
Mapping from Score to PD
-
Adjustment to the PD Economic Cycle
-
Rating Philosophy
-
PD Trough The Cycle (PD TTC) models
-
PD Point in Time PD (PD PIT ) models
-
-
-
PD Calibration of Models Using Machine and Deep Learning
-
Margin of Caution
-
Exercise 29: Modeling the Margin of Caution PD
Module 16: Econometric and AI Models of PD IRB
-
PD estimation
-
Treatment of Panel data
-
Econometric models to estimate PD
-
PD Logistic Regression
-
PD Probit Regression
-
PD COX regression of survival
-
PD Log-log Complementary
-
PD Regression Data Panel
-
PD Bayesian Logistic Regression
-
-
Machine Learning models to estimate PD
-
Cox XGBoost
-
Survival Tree
-
Random Survival Forest
-
Deep Learning Survival
-
PD Neural Networks
-
PD Quantum Neural Networks
-
-
PD Calibration
-
Calibration of econometric models
-
Anchor Point Estimate
-
PD calibration by vintages or vintages
-
Vintage analysis
-
PD Marginal
-
PD Forward
-
Cumulative PD
-
-
Econometric Models
-
Exercise 30: Using COX regression to estimate the PD
-
Exercise 31: Using logistic regression with panel data to estimate PD
-
Exercise 33: Using Bayesian Logistic Regression to estimate PD
-
Exercise 34: Using PD LASSO regression to estimate PD
-
Machine Learning and Quantum Machine Learning
-
Exercise 35: Using Random Forest Survival to estimate PD
-
Exercise 36: Using Cox Xboost to estimate PD
-
Exercise 37: Using Deep Learning survival to estimate PD
-
Exercise 38: Using Feed Forward NN to estimate PD
-
Exercise 39: Using Quantum Neural Networks to estimate PD
Module 17: PD Calibration IRB
-
Concept of adjustment to central tendency
-
Bayesian approach
-
PD calibration in developed countries
-
PD calibration in emerging countries
-
Scaled PD Calibration
-
Scaled Likelihood ratio calibration
-
Smoothing of PD curves
-
Quasi moment matching
-
Approximation methods
-
Scaled beta distribution
-
Asymmetric Laplace distribution
-
-
Rubber function
-
Platt scaling
-
Broken curve model
-
Isotonic regression
-
Gaussian Process Regression
-
Exercise 40: PD calibration using Platt scaling and isotonic regression
-
Exercise 41: PD calibration using Gaussian Process Regression
-
Exercise 42: Calibration of the PD asymmetric Laplace distribution
Module 18: Bayesian PD and Gaussian Process
-
Bayesian and deterministic approach
-
Expert judgment
-
Prior distributions
-
Bayes' theorem
-
Posterior distributions
-
Bayesian PD Estimation
-
Markov Chain–Monte Carlo MCMC approach
-
Credibility intervals
-
Bayesian PD in practice
-
Calibration with Bayesian approach
-
Process Gaussian regression
-
Exercise 43: Logistic Model Bayesian PD in Python
-
Exercise 44: PD using MCMC in R
-
Exercise 45: PD using Process Gaussian Regression
Module 19: Low Default Portfolio PD IRB
-
Confidence interval approach for PD LDP
-
PD estimation without correlations
-
PD estimation with correlations
-
One-period and multi-period estimation
-
-
Bayesian PD estimation for LDP
-
Neutral Bayesian
-
Conservative Bayesian
-
Expert judgment
-
-
Real analysis of PD of Corporate, Sovereign and Retail portfolios
-
LASSO regression to measure corporate default rate
-
Generating synthetic data for LDP using GAN
-
Exercise 46: PD LDP confidence interval approach in R
-
Exercise 47: Multiperiod confidence interval approach PD LDP
-
Exercise 48: Neutral Bayesian PD in R
-
Exercise 49: Conservative Bayesian PD in R
-
Exercise 50: Generating synthetic data with GAN for estimating PD
IFRS 9 PD
Module 20: IFRS 9 PD Forecasting
-
IFRS 9 requirements
-
Probability Weighted Outcome
-
Forward Looking
-
-
Lifetime PD modeling
-
PD Forecasting Modeling
-
PD Point in Time Forecasting
-
PS TTC Forecasting
-
Markov models
-
PIT PD Forecasting Models
-
ARIMA
-
VAR
-
VARMAX
-
ASRF
-
Traditional LSTM
-
Bayesian LSTM
-
Quantum LSTM
-
-
Exercise 51: Forecasting PD using VARMAX in R
-
Exercise 52: PD forecasting using quantum LSTM
Module 21: Lifetime PD
-
Lifetime PD in consumer portfolio
-
Lifetime PD in mortgage portfolio
-
Lifetime PD in Credit Card portfolios
-
Lifetime PD in SMEs portfolio
-
Vintage model
-
Exogenous Maturity Vintage EMV Model
-
Decomposition analysis
-
COVID-19 Pandemic Application
-
Advantages and disadvantages
-
-
Basel ASRF model
-
Matrix ASRF model
-
Leveraging IRB in IFRS 9
-
Advantages and disadvantages
-
-
Regression Models
-
Logistic Multinomial Regression
-
Ordinal Probit Regression
-
-
Survival Models
-
Kaplan–Meier
-
Cox Regression
-
Advantages and disadvantages
-
-
Markov models
-
Multi-State Markov Model
-
Advantages and disadvantages
-
-
Machine Learning Model
-
Support Vector Machine
-
-
Deep Learning Models
-
Neural network architecture
-
-
Lifetime PD Extrapolation Models
-
Lifetime PD Calibration
-
Exercise 53: using multinomial regression for estimating Lifetime PD
-
Exercise 54: using Multi State Markov model for estimating Lifetime PD
-
Exercise 55: using matrix ASRF model for estimating Lifetime PD
-
Exercise 56: using Quantum SVM for estimating Lifetime PD
-
Exercise 57: using traditional SVM in Python for estimating Lifetime PD
-
Exercise 58: using traditional Deep Learning for estimating Lifetime PD
-
Exercise 59: using Quantum Deep Learning for estimating Lifetime PD
IRB LGD
Module 22: LGD IRB in Retail Portfolios
-
Impact of COVID-19 on LGD
-
Definition of default
-
Moratoriums
-
Renovations and restructuring
-
Default Cycle
-
Real Default Cycles
-
-
Expected Loss and Unexpected Loss in the LGD
-
LGD in Default
-
Default Weighted Average LGD or Exposure-weighted average LGD
-
LGD for performing and non-performing exposures
-
Treatment of collaterals in the IRB
-
Workout approach
-
Techniques to determine the discount rate
-
Treatment of recoveries, expenses and recovery costs
-
Default Cycles
-
Recovery expenses
-
-
Downturn LGD in consumer portfolios
-
Downturn LGD in Mortgages
-
LGD in consumption
-
LGD in Mortgages
-
LGD in companies
-
LGD for portfolios with replacement
Module 23: Econometric and machine learning models are used to estimate the Loss Given Default (LGD)
-
Advantages and disadvantages of LGD Predictive Models
-
Forward Looking models incorporating Macroeconomic variables
-
Parametric and non-parametric models and transformation regressions
-
Typology of LGD Multivariate Models
-
Linear regression and Beta transformation
-
Linear Regression and Logit Transformation
-
Linear regression and Box Cox transformation
-
Logistic and Linear Regression
-
Logistic and nonlinear regression
-
Censored Regression
-
Generalized Additive Model
-
Beta regression
-
Inflated beta regression
-
-
Support Vector Regression
-
Support Vector Classification
-
Random Forest Regression
-
XGBoosting Regression
-
Neural networks
-
Deep learning
-
Exercise 60: econometric models for estimating LGD:
-
Logistic and Linear Regression
-
Beta regression
-
-
Exercise 61: Machine Learning and Deep Learning Models for estimating LGD:
-
Random Forest Regression
-
XGBoosting Regression
-
Deep learning Regression
-
-
Exercise 62: Comparison of the performance of the models using Calibration and precision tests.
IFRS 9 LGD
Module 24: LGD for IFRS 9
-
Comparison of IRB LGD vs. IFRS 9
-
Impact on COVID-19
-
IFRS 9 requirements
-
Probability Weighted
-
Forward Looking
-
-
IRB LGD adjustments
-
Selection of Interest Rates
-
Allocation of Costs
-
floors
-
Treatment of collateral over time
-
Duration of COVID-19
-
-
LGD PIT modeling
-
Collateral Modeling
-
LGD IFRS 9 for portfolio companies
-
LGD IFRS 9 for mortgage portfolio
-
LGD IFRS 9 for corporate portfolios
-
IFRS 9 LGD using LASSO Regression
-
Machine Learning Models
-
Support Vector Machine
-
Random Forest
-
Xgboost
-
Neural Networks
-
-
Exercise 63: Estimation and adjustments for LGD IFRS 9 using Random Forest regression
-
Exercise 64: Estimation and adjustments for LGD IFRS 9 Beta Regression and Neural Networks
-
Exercise 65: Estimating IFRS 9 LGD using Xgboost regression in Python
-
Exercise 66: Estimating IFRS 9 LGD using traditional SVM
-
Exercise 67: Estimating IFRS 9 LGD using quantum SVM
-
Exercise 68: Estimating IFRS 9 LGD using traditional neural networks
-
Exercise 69: Estimating IFRS 9 LGD using Bayesian neural networks
IRB EAD
Module 25: Development of models to estimate the Exposure at Default (EAD) and the Credit Conversion Factor (CCF) for the Internal Ratings-Based (IRB) approach
-
Impact of COVID-19 on credit lines
-
Guidelines for estimating CCF
-
Guidelines for Estimating CCF Downturn
-
Temporal horizon
-
Transformations to model the CCF
-
Approaches to Estimating CCF
-
Fixed Horizon approach
-
Cohort Approach
-
Variable focus time horizon
-
-
Econometric Models
-
Beta regression
-
Inflated beta regression
-
Fractional Response Regression
-
Mixed Effect Model
-
-
Machine Learning Models
-
Neural networks
-
SVM
-
-
Intensity model to measure the withdrawal of credit lines
-
Exercise 70: Neural network regression model for estimating CCF
-
Exercise 71: Support Vector Regression Model in Python for estimating CCF
-
Exercise 72: Neural Networks and beta regression in R for estimating CCF
-
Exercise 73: Quantum SVM and Classic Regression SVM for estimating CCF
IFRS 9 EAD
Prepayment Rate
Module 26: Contractual Options
-
Prepayment and other options
-
IFRS 9 requirements
-
Probability Weighted
-
Forward Looking
-
IFRS 9 prepayment modeling
-
Cox Regression
-
Logistic regression
-
-
Survival rate estimate
-
Joint Probability Model with PD Lifetime
-
Exercise 74: prepayment model based on random forest regression, implemented using R and Excel.
Module 27: Lifetime EAD for Credit Lines
-
The pandemic has significantly affected the utilization of credit lines.
-
Lifetime measurement in credit cards
-
Lifetime EAD
-
IFRS 9 requirements
-
Probability Weighted
-
Forward Looking
-
Adjustments in the EAD
-
Interest Accrual
-
CCF PIT Estimate
-
CCF Lifetime Estimate
-
EAD lifetime modeling
-
Model of the use of credit lines with macroeconomic variables
-
Credit card abandonment adjustment
-
EAD Lifetime model for pool of lines of credit
-
vintage model
-
Chain Ladder Approach
-
-
Exercise 75: Neural network model for line of credit
-
Exercise 76: EAD Lifetime model for line of credit
BACKTESTING VALIDATION
Module 28: PD Backtesting
-
Validation of PD
-
Backtesting of PD
-
Statistical test:
-
Hosmer Lameshow test
-
Normal test
-
Binomial Test
-
Spiegelhalter test
-
Redelmeier Test
-
Traffic Light Approach
-
-
Traffic Light Analysis and Dashboard
-
Stability Test for PD
-
Comparing Predicted Probability of Default (PD) with Default Rate over Time.
-
Performing a validation through Monte Carlo simulation
-
Exercise 77: Backtesting PD in Excel
Module 29: LGD Backtesting
-
LGD Backtesting
-
Accuracy ratio
-
Absolute accuracy indicator
-
Confidence Intervals
-
Transition analysis
-
RR Analysis using Triangles
-
Advanced LGD Backtesting with a vintage approach
-
Backtesting for econometric models:
-
Test calibration
-
T-test
-
Wilcoxon signed rank test
-
Accuracy Test
-
F Test
-
Ansari–Bradley Test
-
Exercise 78: Comparison of the performance of the models using Calibration and precision tests.
Module 30: EAD Backtesting
-
EAD Performance
-
R squared
-
Pearson coefficient
-
Spearman correlation
-
Validation using ROC, KS and Gini
-
Exercise 79: Comparison of the performance of EAD models
STRESS TESTING IRB
Module 31: Deep Learning for Modeling Macroeconomic Dynamics
-
Macroeconomic models
-
Neoclassical growth model
-
Partial differential equations
-
DSGE Stochastic Dynamic General Equilibrium Models
-
Deep learning architectures
-
Reinforcement Learning
-
Advanced Scenario Analysis
-
Exercise 80: using neural networks for Bellman equation macroeconomic model
Module 32: Deep Learning models for macroeconomic projections
-
Multivariate Models
-
VAR Autoregressive Vector Models
-
ARCH models
-
GARCH models
-
GARCH Models Multivariate Copulas
-
VEC Error Correction Vector Model
-
Johansen's method
-
-
Machine Learning Models
-
Supported Vector Machine
-
Neural network
-
Forecasting market time series yields
-
NN and SVM algorithms for performance forecasting
-
Forecasting volatility NN vs. Garch
-
-
Development and validation base
-
-
Deep learning
-
Recurrent Neural Networks RNN
-
Elman's Neural Network
-
Jordan Neural Network
-
Basic structure of RNN
-
Long short term memory LSTM
-
temporary windows
-
Development and validation sample
-
Regression
-
Sequence modeling
-
-
Quantum Deep Learning
-
Time series analysis with Facebook Prophet
-
Prediction of the spread of Covid-19
-
Exercise 81: Charge-off model with VAR and VEC
-
Exercise 82: Forecasting PD using financial series and Bayesian LSTM indices in Python
-
Exercise 83: Pandemic Forecasting using Multivariate RNN LSTM in Python
-
Exercise 84: Forecasting PD using quantum neural networks
Module 33: Stress Testing PD and LGD
-
Temporal horizon
-
Multi-period approach
-
Data required
-
Impact on P&L, RWA and Capital
-
Macroeconomic Stress Scenarios in consumption
-
Expert
-
Statistical
-
Regulatory
-
-
Stress Testing PD :
-
Credit Portfolio View
-
Multiyear Approach
-
Reverse Stress Testing
-
Rescaling
-
Cox Regression
-
-
Stress Testing of the Transition Matrix
-
Credit Portfolio View Approach
-
Credit cycle index
-
Multifactor Extension
-
-
Stress Testing LGD:
-
Downturn LGD : Mixed Distribution Approach
-
PD/LGD Multiyear Approach modeling
-
LGD stress test for mortgage portfolios
-
-
Stress Testing of:
-
Net Charge Off
-
Rating/Scoring transition matrices
-
Recovery Rate and LGD
-
-
Exercise 85: using Credit Portfolio Views for Stress Testing PD
-
Exercise 86: using Bayesian LSTM for Stress Testing PD
-
Exercise 87: using Variational Quantum Regression for Stress Testing PD
-
Exercise 88: using MARS Model for Stress Testing PD
-
Exercise 89: using LASSO regression for Stress Testing PD
Module 34: Stress Testing in corporate portfolios
-
Temporal horizon
-
Data required
-
Main Macroeconomic variables
-
Impact on P&L, RWA and Capital
-
ASRF model
-
Creditmetrics model
-
Using Transition Matrices
-
Use of the credit cycle index
-
Default forecasting
-
Stress Test Methodology for corporate portfolios
-
Impact on RWA and Capital
-
Exercise 90: Performing stress tests for Probability of Default (PD) in corporate portfolios using a transition matrix and the ASRF model
STRESS TESTING ECL IFRS 9
Module 35: Stress Testing under ECL IFRS 9
-
Stress testing under IFRS 9 and COVID-19
-
Pandemic scenarios applied to the ECL calculation
-
Stress Testing of IFRS 9 parameters
-
EBA Stress Testing 2023
-
Treatment of the moratorium
-
Possible regulatory scenarios
-
Impact on P&L
-
PIT starting parameters
-
PIT projected parameters
-
Calculation of non-productive assets and impairments
-
Changes in the stock of provisions
-
Changes in the stock of provisions for exposures phase S1
-
Changes in the stock of provisions for exposures phase S2
-
Changes in the stock of provisions of exposures phase S3
-
Sovereign Exposure Impairment Losses
-
Impact on capital
-
Internal Stress Testing Model for ECL IFRS 9
-
Stage Migration
-
Stage Transition Matrix
-
Stress testing of PDs and credit migrations
-
Stress testing of exhibitions
-
Stress testing of recoveries
-
Exercise 91: An internal global exercise was conducted for ECL stress testing using R and Excel
Module 36: Quantum Stress Testing
-
Quantum economics
-
Classic Monte Carlo simulation
-
Quantum Monte Carlo
-
Coding Monte Carlo problem
-
Breadth Estimation
-
Acceleration applying the amplitude estimation algorithm
-
DGSE model using neural networks
-
Quantum Monte Carlo Simulation vs Normal Monte Carlo Simulation
-
Exercise 92: DGSE model using deep learning
-
Exercise 93: Quantum Monte Carlo Simulation vs. Classical Monte Carlo Simulation
CREDIT RISK PORTFOLIO
Module 37: Economic Capital Models
-
Regulatory Capital
-
Economic Capital Methodologies
-
Correlation of Assets and Default
-
Unexpected Tax Loss
-
ASRF Economic Capital Models
-
Business Models
-
Kmv
-
creditmetrics
-
Credit Portfolio View
-
Credit risk +
-
-
Economic capital management
-
Allocating Economic Capital
-
Exercise 94: Portfolio Approach: Estimation of EL, UL, ULC, Correlation and Economic Capital in Excel
-
Exercise 95: Creditrisk + in SAS
-
Exercise 96: Creditmetrics in Excel and R
-
Exercise 97: Single-factor model in Excel
QUANTUM COMPUTING FOR ECONOMIC CAPITAL
Module 38: Quantum computing for Economic Capital
-
Distribution of credit risk losses
-
Quantum uncertainty model
-
Circuit Definition
-
Quadratic acceleration over the classical Monte Carlo simulation
-
Expected loss
-
Cumulative distribution function
-
VaR
-
Expected Shortfall
-
Exercise 98: Estimation EL, VAR, ES of quantum credit risk
Quantifying climate change-related credit risk
Module 39: Climate Risk in Credit Risk
-
Credit Risk Transition risk
-
Scenario analysis to assess the transition risk component of a portfolio's credit risk
-
Introduction: preparing banks for the low carbon transition
-
A growing need for climate scenario analysis
-
The challenge for banks
-
Take advantage of and integrate the resources available to banks
-
-
An integrated approach to transition risk assessment Transition scenarios
-
Understand transition scenarios and their sources
-
Using scenarios for transition risk assessment
-
Closing the gap between climate scenarios and financial risk assessment
-
-
Borrower Level Calibration
-
Portfolio Impact Assessment
-
Link expected loss to transition impacts on portfolios
-
Assessment of probability of default (PD)
-
Loss Given Default (LGD) Assessment
-
-
Putting the Approach to Work: Lessons Learned from Banking Pilots
-
Piloting the transition risk methodology
-
Definition of sectors and segments
-
Evaluate the relative sensitivities of the segments
-
Determination of calibration points at the borrower level Case studies and results
-
The pilot transition scenario
-
pilot results
-
-
Transition Opportunities: Exploring an Institutional Strategy
-
evaluating the market
-
Grounding Opportunity Assessments in Scenario Analysis
-
Assessing the market attractiveness of the segment
-
Identification of banking capabilities
-
Discovering the opportunities with the greatest potential
-
-
Future Directions: Developing the Next Generation of Transition Risk Analysis
-
-
Physical risks and opportunities
-
An Integrated Approach to Physical Risk Assessment
-
Borrower Characteristics
-
Insurance as a risk mitigator due to extreme climatic and meteorological events
-
climate change scenarios
-
-
Impacts of climate change on the probability of default PD
-
Evaluation of changes in the productivity of the sector
-
Adjustment of income statement metrics
-
Determination of changes in the probability of default
-
-
Real Estate: Climate Change Impacts on LTV Loan-to-Value
-
Estimation of the impacts of extreme events on the value of properties.
-
Determining Changes in LTV Loan-to-Value Ratio
-
-
Physical Opportunities: Exploring an Institutional Strategy
-
Taxonomy of opportunities and data sources
-
evaluating the market
-
Evaluation of the financing demand of the sector
-
Sector evaluation
-
Assess the institutional capacity and market positioning of a bank
-
Evaluate opportunities
-
-
Future Directions: Towards the Next Generation of Physical Risk and Opportunity Analysis
-
Develop internal analytics and capabilities within banks
-
Strengthening the research base
-
Develop analytical platforms and tools to support physical assessments of risks and opportunities.
-
Improve information flows on physical risk and adaptation between banks and borrowers
-
Improve dialogue with governments and insurers
-
-
-
Exercise 99: Estimating PD and DD adjusted for climate change in transition risk
-
Exercise 100: Estimating PD and DD adjusted for climate change in physical risk